What is Confidence/Uncertainty in a Price? By Pyth
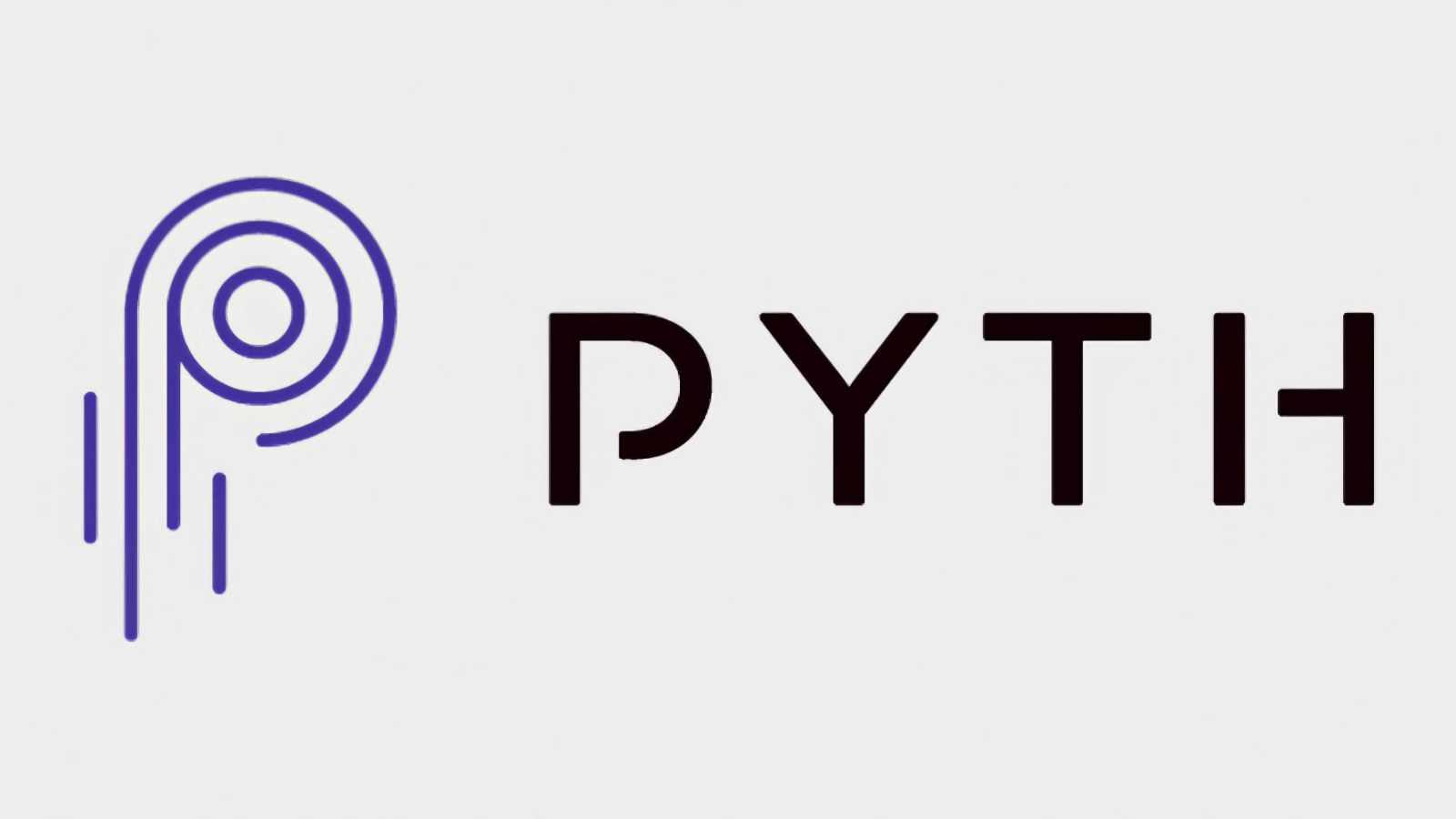
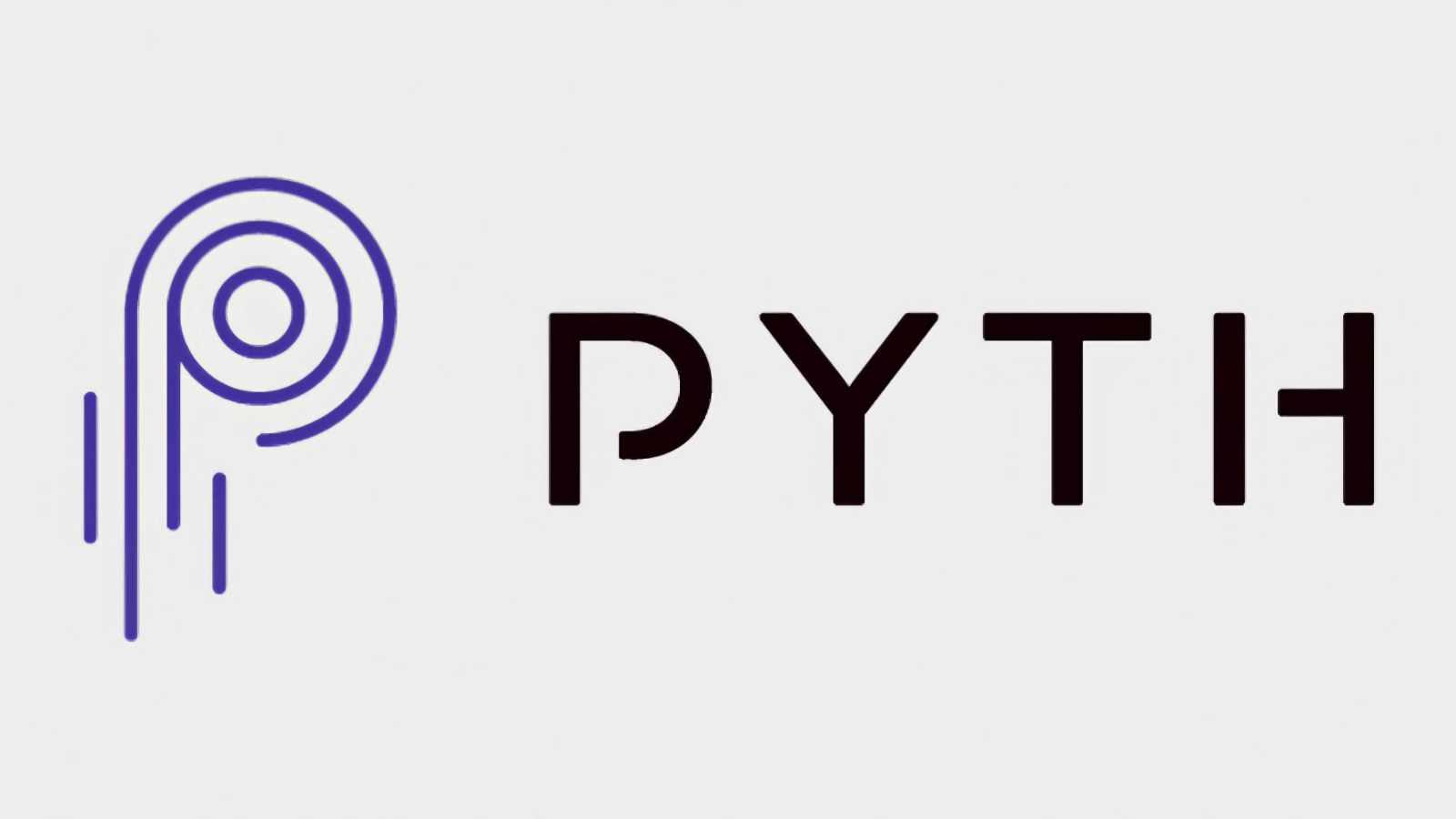
Pyth Network talked confidence and empowering data consumers by continuously publishing a consolidated estimate of important dislocations.
- Pyth data providers stream a price along with the confidence/uncertainty that they estimate using information available to them.
- For example, a data provider focused on a particular market could represent confidence as the bid / ask spread on that venue.
- Alternatively, focused on multiple venues, a data provider could represent confidence as a function of where they expect trades to occur.
- In different markets, uncertainties will vary due to market structure.
Measurement Uncertainty
In scientific and engineering fields, an observation or measurement is almost always accompanied by a measurement uncertainty. The distance between two points might be measured to be 10.12m +/- 0.01m. The time it took for a car to travel that distance might be measured to be 1.23s +/- 0.05s. The measurement uncertainty is the observer’s best estimate of how far off from the “true” value their measurement is likely to be, given the precision of their measuring equipment, the difficulty in making the measurement, and potentially other factors the observer deems important.
Why Do Measurement Uncertainties Matter?
- It indicates how much precision or uncertainty there is in a single observation.
- It allows one to combine multiple independent observations of the same thing in a more “optimal” way, giving more weight to observations with smaller uncertainty and less weight to observations with greater uncertainty.
- When measurements are combined to make derived quantities (distance and time measurements in the example above combined to derive a speed estimate, for example), uncertainties in the raw measured values can be propagated to make uncertainties in the derived quantities (speed estimate in this example would be 8.2+/- 0.3 m/s).
- Independent measurements of the same thing that do not agree with each other to within their respective uncertainties are an indication of a problem with one or more of the measurements.
How does this relate to a Pyth data provider’s price and uncertainty?
Suppose quoter A exclusively uses data from one of the US exchanges, and they are basing their Pyth quote on the order book of that exchange. They may well decide that their best estimate of TSLA price is the midpoint of that exchange’s bid and ask price, with an uncertainty of half the bid-ask spread. If the best bid on that exchange was $10.25 and the best ask was $10.35, they would report TSLA price as $10.30 +/- $0.05.
Suppose quoter B is using data exclusively from a different exchange, where TSLA is more heavily traded and the bid-ask spread is narrower. If that bid was $10.26 and that best ask was $10.28, they would report the TSLA price as $10.27 +/- $0.01.
Suppose quoter C is a proprietary trading firm that actively trades TSLA on many different trading venues. They might be constructing their estimate of TSLA price based on the prices of their own trades at all venues. For example, they may be buying at a price of $10.27 on exchange D (who is not a quoter) and selling at a price of $10.265 (via hidden midpoint orders on a dark pool that is also not a quoter) and report a TSLA price of $10.2675 +/- $0.0025.
Suppose quoter D is also a proprietary trading firm, but they are not as active in TSLA. If their last trade in TSLA was 15s ago at a price of $10.19, they might take their estimate of TSLA’s price volatility and increase their quote’s uncertainty like the sqrt of time since their last trade (treating price like a random walk). They might report a TSLA price of $10.19 +/- 0.03.
All of these examples show how individual quoters might derive both a price and an uncertainty from the particular data that they have access to in the case of US equities. You will notice as well that the quotes are all consistent with each other (i.e., the difference between quotes is not big compared to the uncertainties of the quotes). The Pyth aggregation algorithm combines these quotes on-chain and gives more weight to the quotes with smaller uncertainties.
FX Markets
Unlike US equity markets (which are “all to all markets” meaning that everyone’s orders can match with everyone else’s orders and there is at least a single notion of “best price”), many FX markets are bilateral or multilateral markets, where participants can specify which counterparties they are willing to trade with (or which ones they are not willing to trade with). This results in an even more complex “fragmented market” where there is not an accepted measurement of “best price”.
Crypto Markets
Unlike the highly regulated US equity market, global crypto markets are not required to route orders to better priced markets. Different exchanges can and do “cross” each other often, with the best bid price on exchange A being higher than the best ask price on exchange B. Furthermore, because trading fees can be quite significant, these price differences can and do persist for substantial periods of time, because they cannot be arbitraged away due to the high fees. If Bitcoin is trading (bid, ask) at (30,000.00, 30,000.05) on market A, but simultaneously (and for an extended period) trading 1.5 bps higher on market B with a (bid, ask) at (30,005.00, 30,005.05), then what is “the price of Bitcoin”? In a very real sense, there is a ~$5 uncertainty in the price of Bitcoin, because it is trading simultaneously at two different exchanges at prices that are $5 apart from each other!
What is Pyth?
Smarter data for smarter contracts. Pyth is designed to bring real-world data on-chain on a sub-second timescale.
📰 INFO:
https://pythnetwork.medium.com/what-is-confidence-uncertainty-in-a-price-649583b598cf